Stanford study showing widespread infection in Santa Clara county has many problems
Follow @PapersCovidYou probably saw the headline
related to a recent preprint. The conclusion that many have drawn is that we can conclude that COVID-19 is far less deadly than believed. However, the study is flawed in many ways.
Stanford Serology study preprint just posted that is certain to mislead many people:https://t.co/eitVtfjYoP
— A Marm Kilpatrick (@DiseaseEcology) April 17, 2020
It's a serological study, which is fantastic. We need these kinds of studies and data badly. Unfortunately this paper is badly misleading (bordering on purposeful?)
The basic flow of the study was that the researchers posted Facebook ads to recruit participants
Respondents were brought into the lab and tested, with 1.5% testing positive. They then did some statistical adjustments which you can read more about in an analysis by Andrew Gelman here
Here are some of the problems:
Selection bias: many problems with recruiting participants the way they did, two highlighted below
It recruits people via Facebook ads which are clearly not a random sample of the population. The most important subject trait given the influence of age on COVID-19 mortality is: AGE! And yet this study does not present seroprevalence by age or adjust its estimates by age.
— A Marm Kilpatrick (@DiseaseEcology) April 17, 2020
The Stanford COVID-19 serology study that's beginning to make rounds has some serious polling methodology problems. The biggest of which is that the way that it solicited respondents. You can't mention COVID-19 in the ad and get an accurate sample https://t.co/y56hrAbO4D pic.twitter.com/1sOVQVCGLv
— Matthew Sheffield (@mattsheffield) April 17, 2020
Results are highly sensitive to estimates of the test’s accuracy: This is related to Bayes’ theorem, in short if you are testing for something that is even a little rare, you need to have a far higher test specificity than intuition would suggest. The probability that someone has a rare condition because even a highly accurate test says so can still be very very low.
Borrowing an example from Wikipedia: supposing you’re trying to detect drug use with a test that’s 99% sensitive and specific. If only 0.5% of people are drug users, the probability that someone is a drug user given that they fail the drug test is…….33.2%.
Stanford serosurvey is getting lots of attention. But it warrants caution. They are making bold claims (50x undercounting of infections) assuming a test specificity of 99.5%. If that's off by even a tiny bit, undercounting estimate approaches 0. https://t.co/1eUDOPgVwH
— mbeisen (@mbeisen) April 17, 2020
The authors themselves acknowledge that their results would evaporate pretty readily if their estimate of the test’s accuracy is off by even a bit
They note this in the paper : "For example, if new estimates indicate test specificity to be less than 97.9%, our SARS-CoV-2 prevalence estimate would change from 2.8% to less than 1%, and the lower uncertainty bound of our estimate would include zero."
— mbeisen (@mbeisen) April 17, 2020
Statistical corrections seem flawed in obvious ways: Since they knew their sample of participants from Facebook wasn’t representative they attempted to correct for this using a statistical technique. The problem is that their final corrected dataset isn’t corrected for age and is largely skewed as a result.
A few other problems noted here
Take a closer look at the new #covid19 #serology study by @Stanford team (preprint):
— Simone Toppino (@SimoneToppinoMD) April 18, 2020
- Fb-based sample selection
- mostly young females
- no adjustment nor stratification for age
- no data on symptoms
- no parallel PCR testing
Watch out for easy conclusions! https://t.co/v5kpqXnH57
It’s worth noting that two of the study’s authors penned a WSJ op-ed recently showing their guess that
current estimates about the Covid-19 fatality rate may be too high by orders of magnitude.
Though they found this result, they had to make a lot of basic mistakes to do so.
As Gelman concludes
I think the authors of the above-linked paper owe us all an apology. We wasted time and effort discussing this paper whose main selling point was some numbers that were essentially the product of a statistical error.
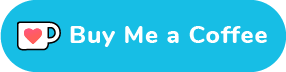